Image description: "The assessment of potential societal risks brought about by artificial intelligence (AI)" is a sentence I asked an AI to visualise. An alluring image of a female robot has been crafted.
Computer Engineering (Hons), a writer for GABEY, primarily focuses on cybersecurity and the changing nature of online threats.
In this series of three posts, we explore the risks associated with using computers to make decisions that could potentially result in fatalities.
Part 1
In today's rapidly advancing technological landscape, delegating life-or-death decisions to algorithms has become a thought-provoking question. Entrusting digital entities with the power to determine human fate may seem like science fiction, but with progress in artificial intelligence and machine learning, it's increasingly possible. Advocates argue that algorithms lack emotions and biases, making them objective decision-makers.
This post series, consisting of three parts, explores the dangerous risk of using algorithms to make decisions that could result in fatal outcomes. The complexity of this moral dilemma has perplexed humanity for centuries, highlighting the challenge of finding a resolution through computer calculations alone. In this era of advancing technology, retaining human control over decisions is crucial, despite technology's growing role in decision-making processes.
Algorithms, from social media platforms to insurance policies, have become essential in shaping our daily lives. These complex mathematical models revolutionize decision-making by providing tailored experiences and individualized suggestions through vast data analysis.
In the insurance industry, algorithms assess risk and set premiums with unprecedented accuracy by analyzing vast datasets. This enables insurers to provide precise evaluations and customized coverage to clients.
While algorithms offer efficiency and convenience, ethical considerations are paramount. Their dependability hinges on data quality and adherence to regulations.
Decision-Making Algorithms: A Brief History
Algorithmic decision-making has been around for several centuries, and its evolution is closely tied to advancements in mathematics, technology, and computing. In this section, we will explore critical milestones in the historical development of this process.
Algorithms have been around since ancient times. The Greeks, for example, used algorithms to determine prime numbers and find the greatest common divisor. The algorithm created by Euclid in the third century BCE is still relevant in number theory and continues to be used today.
During the mid-20th century, the advancement of modern computing elevated algorithmic decision-making to a new level. Alan Turing, a renowned mathematician, established the basis for modern computers with his work on the Turing Machine. Additionally, his widely-known "Turing Test" delved into the concept of machine intelligence.
During the 1950s and 1960s, computer algorithms were devised to primarily focus on handling rudimentary tasks, such as organising and retrieving data through sorting and searching operations. For example, the "Bubble Sort" algorithm demonstrated proficiency in managing data sets, whereas the "Binary Search" algorithm facilitated the identification of particular items within sorted arrays.
In the 1970s and 1980s, artificial intelligence experienced significant advancements that led to the development of expert systems. These systems utilised rule-based algorithms to imitate the decision-making abilities of human experts in specific fields. Among the most renowned examples of such systems is the MYCIN system, which demonstrated the ability to diagnose infectious diseases with a similar level of accuracy as human experts. This breakthrough in the application of AI technology was a major milestone in the field, marking a significant step towards creating computer systems that can match or even surpass the capabilities of human experts in various domains.
In the latter part of the 20th century and the beginning of the 21st century, a marked surge in the application of machine learning algorithms that learned from data rather than being specifically programmed was observed. Some notable examples of these algorithms are decision trees, neural networks, and support vector machines. Implementing these algorithms has paved the way for data-driven decision-making in many sectors, such as image recognition, natural language processing, and recommendation systems.
The prevalence of algorithms has brought attention towards the issue of bias and fairness. Discrimination caused by algorithms in hiring, lending, and criminal justice has raised ethical and social concerns. However, researchers and policymakers are actively working towards developing fairer algorithms and reducing bias.
Social media platforms heavily depend on algorithms to cater to individual user experiences. For example, Facebook's news feed algorithm selects content based on users' interactions, preferences, and behaviour, which ultimately determines the information displayed to the users.
The financial industry has been revolutionised by algorithmic trading, which has transformed the process of stock market transactions. High-frequency trading algorithms can execute trades at lightning speed, allowing them to respond quickly to market conditions and seize fleeting opportunities.
In recent years, the development of self-driving cars has reached new heights, thanks to machine learning and computer vision. These vehicles utilise advanced algorithms to analyse sensor data and make swift decisions to navigate on roads safely.
Machine learning algorithms have proven to be a promising tool in the healthcare industry, particularly in disease diagnosis and treatment planning. By analysing medical imaging data, these algorithms can effectively detect anomalies and provide medical professionals with the necessary support to make accurate diagnoses.
Algorithms significantly impact multiple fields, from traditional mathematical principles to modern AI advancements. We should acknowledge their potential to revolutionize decision-making and shape the technological landscape.
Algorithmic Bias
Algorithmic bias is a highly consequential risk that warrants attention. The effectiveness of algorithms is contingent upon the data quality they are trained on. Should the data contain biases, the algorithms will acquire and perpetuate those biases. There have been several significant occurrences of algorithmic bias observed across various domains. Algorithmic bias refers to the phenomenon where computer algorithms generate outcomes that consistently exhibit preferential treatment or discrimination towards specific demographic groups, considering attributes such as race, gender, age, or other legally protected characteristics.
It has been observed that facial recognition systems may demonstrate biases related to race and gender, resulting in the potential for misidentification or inaccuracies when processing data for individuals belonging to certain ethnicities or genders. The presence of this bias has generated apprehension regarding the possibility of discriminatory practices within the realms of law enforcement and surveillance.
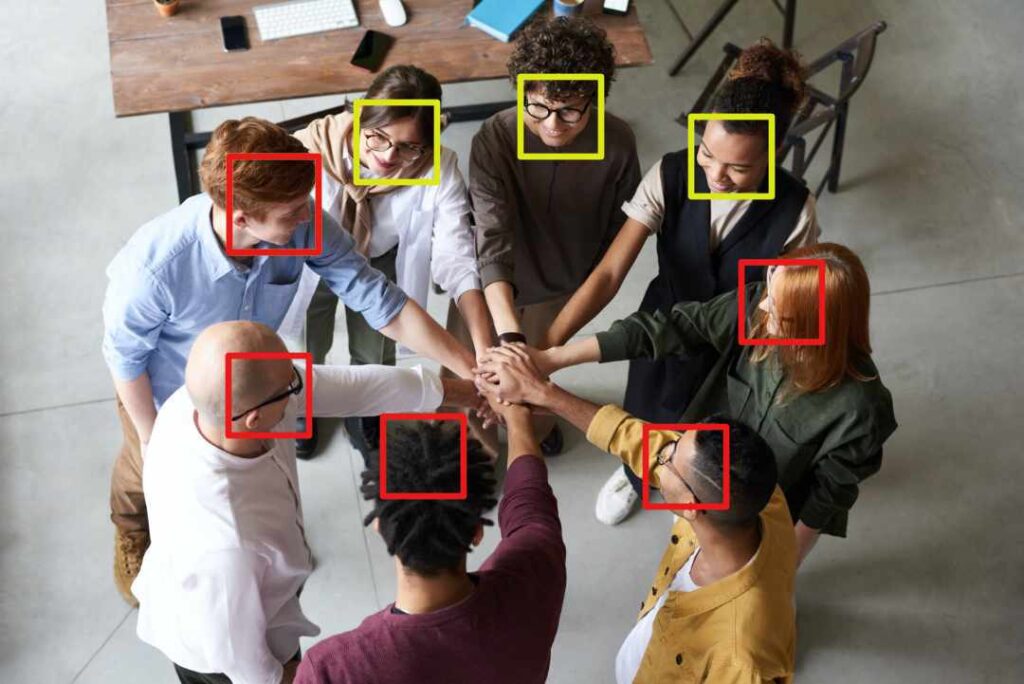
There have been previous concerns regarding potential bias in Amazon's facial recognition software, Rekognition. According to a study conducted by the Massachusetts Institute of Technology in 2019, it was determined that Rekognition exhibited a 31% error rate in accurately identifying the gender of images depicting women with dark skin. In light of these concerns, Amazon made an announcement in 2020 regarding implementing a one-year moratorium on utilising Rekognition by law enforcement agencies.
It has been discovered that specific hiring algorithms can contribute to gender and racial biases when assessing job candidates. For instance, if an analysis of past hiring data reveals a tendency for hiring algorithms to exhibit bias towards certain demographic groups, there is a risk that the algorithm will perpetuate this bias and result in discriminatory outcomes in subsequent hiring processes. This can result in particular demographic groups being given preferential treatment, which is unfair for hiring.
In 2015, researchers from the University of Washington discovered a notable underrepresentation of women in the image results when searching for various occupations, such as "CEO." Furthermore, the researchers observed that these search results have the potential to influence the perspectives of individuals conducting the searches. Subsequently, Google has asserted that it has resolved this matter. According to a paper presented at the AAAI Conference of Artificial Intelligence in February, the researchers demonstrated that the bias in four prominent search engines, including Google, has only been partially addressed.
Credit scoring algorithms have been criticised for potentially having racial bias when determining creditworthiness. Research has demonstrated that certain credit-scoring models may unjustly penalise minority groups, causing difficulty obtaining loans or credit.
Algorithmic tools utilised in criminal justice, such as risk assessment algorithms, have been observed to manifest racial bias, resulting in disparate treatment of individuals in the context of sentencing and parole determinations.
The algorithms employed by social media platforms to curate content and provide recommendations for users have the unintended consequence of reinforcing echo chambers and promoting biased content. This, in turn, contributes to the spread of misinformation and exacerbates polarisation among users.
The Guardian's article, "'There is no standard': investigation finds AI algorithms objectify women's bodies," sheds light on an analysis that reveals the bias of AI algorithms used by social media platforms to moderate content towards women's bodies. The study discovered that these algorithms are more likely to label pictures of women in everyday situations as "racy" or "sexually suggestive" than those of men in similar circumstances. This bias results from the algorithms being trained on data favouring men's bodies over women's.
The article also highlights the negative impact of this bias on women, including censorship on social media and increased self-consciousness about their bodies.
Addressing algorithmic bias is critical to ensure fairness, transparency, and accountability in automated decision-making systems. Researchers, policymakers, and organisations are making a concerted effort to develop and deploy algorithms that are free from bias and that promote equitable outcomes for all individuals. Some strategies being explored to mitigate algorithmic bias include regular audits, diverse algorithm development teams, and ongoing monitoring.
Criminal Justice Algorithms
Criminal justice systems frequently employ algorithms to assist in determining sentencing and parole rulings. However, these algorithms have been found to possess biases that can lead to unjust treatment, particularly for underrepresented groups. In some cases, these algorithms may even contribute to the continuation of racial inequalities present within the criminal justice system.
The criminal justice system relies increasingly on algorithms to anticipate the possibility of repeated criminal offences. One algorithm in particular, COMPAS (Correctional Offender Management Profiling for Alternative Sanctions), evaluates the likelihood of recidivism through an interview with the defendant and their past criminal record.
Nevertheless, there are apprehensions regarding the utilisation of algorithms to forecast recidivism. One issue is the potential bias in the training data utilised for these algorithms, which can result in subjective predictions. If the dataset used to train the algorithm exhibits a disproportionate representation of specific demographic groups, the algorithm may generate biased predictions about said groups.
One issue with algorithms is that they may need help fully understand and account for human behaviour and decision-making complexity. For instance, a person's probability of committing another crime could be influenced by various factors such as their social support system, access to resources, and personal situation. It can be challenging for an algorithm to consider and include all these factors when predicting outcomes accurately.
Overreliance on Algorithms
The excessive dependence on algorithms may reduce the significance of human judgement and decision-making. Making decisions based only on algorithms and subjecting them to rigorous analysis can result in well-informed decisions and less dependence on human intervention.
Social Media Content Curation
Social media platforms heavily rely on algorithms to personalize user experiences. Facebook, Twitter, and Instagram are social media platforms that use sophisticated algorithms to personalize users' news feeds by displaying content based on their interactions and interests. They analyse browsing history, likes, and interactions to curate news feeds and suggest content matching users' interests. Social media platforms use advanced algorithms to shape users' experiences by predicting their preferences before they know them. These platforms customise news feeds and suggest content according to users' interactions and preferences. However, excessive reliance on these algorithms can lead to echo chambers, where users are only exposed to viewpoints that support their existing beliefs, thus limiting their exposure to diverse perspectives. Such algorithms may contribute to social divisions and limit exposure to diverse perspectives.
Online Recommendations
Online shopping sites, streaming services, and content platforms heavily depend on algorithms to offer tailored suggestions to their users. Although this can improve the user experience, it may also reduce options and convergence of preferences, restricting users' access to various and fresh content. E-commerce platforms use recommendation algorithms to provide personalised product suggestions to customers, considering their browsing history and purchasing behaviour. These algorithms can improve user experiences, boost customer satisfaction, and drive sales. These advanced algorithms can customise experiences and recommendations according to individual preferences and behaviours. E-commerce platforms leverage recommendation algorithms to suggest products that align with users' interests, resulting in heightened customer satisfaction and boosted sales.
These are some online shopping sites, streaming services, and content platforms that heavily rely on algorithms to provide personalised suggestions to their users:
Amazon utilises sophisticated algorithms to provide personalised product recommendations to users, leveraging their previous purchase history, browsing activities, and ratings. For instance, if you have recently purchased a book on the Amazon platform, the website might provide recommendations for additional books authored by the same writer or falling within the same genre.
Netflix utilises advanced algorithms to provide users with personalised recommendations of films and TV shows. These recommendations are based on a comprehensive analysis of the user's viewing history, ratings, and preferences of other users with similar viewing habits. For instance, if you have recently viewed a comedy on Netflix, the platform might suggest other comedies or highly-rated shows favoured by fellow comedy enthusiasts.
Advanced algorithms, such as collaborative filtering, provide personalised recommendations to their users. Collaborative filtering gathers information from users with similar preferences to predict a user's interests. The algorithm then identifies patterns in the data to make recommendations to individual users.
The collaborative filtering algorithm analyses millions of users' viewing history and behaviour to identify patterns and relationships between movies and TV shows. When many users who watched a particular film also watched another, the algorithm infers a relationship between the two films and recommends the second movie to users who have watched the first but not the second. By using collaborative filtering, Netflix can provide highly personalised recommendations to its users, helping them discover new content they are likely to enjoy. This improves the user experience and allows Netflix to retain subscribers by providing a steady stream of relevant and engaging content.
YouTube utilises algorithms to provide video recommendations to users, considering their viewing history, preferences expressed through likes or dislikes, and the popularity of videos among other users. For instance, if you have recently viewed a video about felines, YouTube may suggest additional videos related to cats or videos that have garnered significant attention from fellow enthusiasts of feline companions.
Spotify utilises algorithms to provide music recommendations to users, considering their listening history, followed artists, and popular songs among other users. For instance, if you have recently engaged with a musical composition by the renowned artist Taylor Swift, Spotify can suggest additional tracks by Taylor Swift or songs that have garnered significant popularity among other Taylor Swift enthusiasts.
These algorithms are continually improving and adapting, resulting in more personalised user recommendations as time goes by.
Hiring and Recruitment
Many companies utilize algorithms for their hiring and recruitment procedures, which assist in sifting through resumes and pinpointing promising candidates. Even so, excessive trust in algorithms can result in excluding eligible candidates who fail to meet specific keywords or criteria. This could foster biases and restrict workplace diversity.
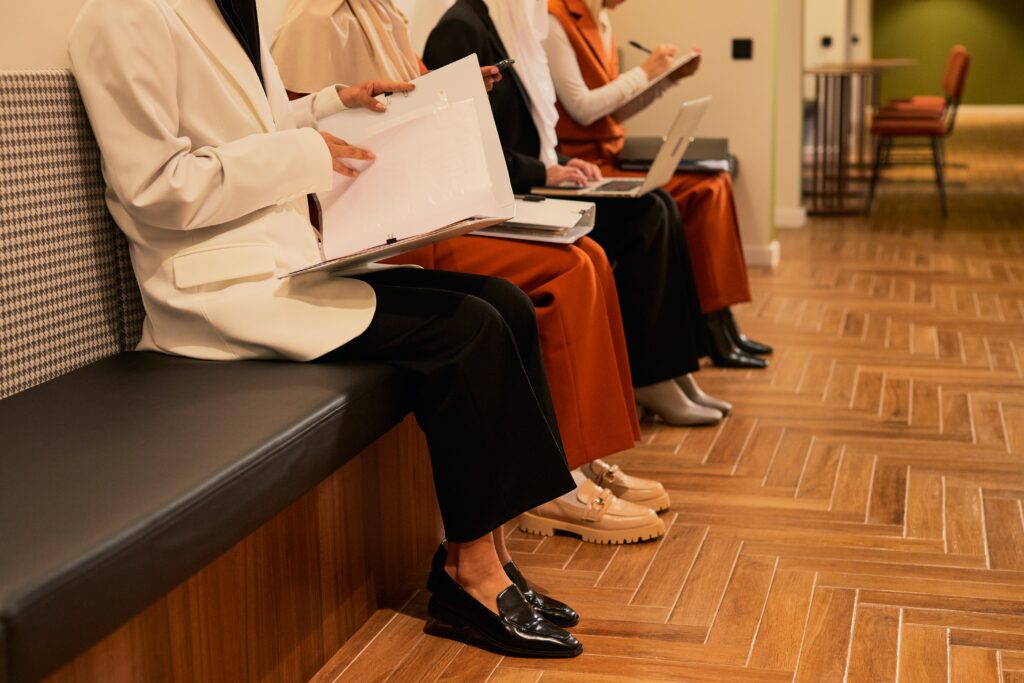
The growing dependence on algorithmic decision-making presents both opportunities and potential risks. Although algorithms have demonstrated their efficacy in diverse fields, they possess inherent risks that can yield substantial consequences. Let us now examine a few instances that illustrate the potential hazards associated with algorithmic decision-making:
One of the most significant dangers is algorithmic bias. Algorithms are only as good as the data they are trained on, and if the data contains preferences, the algorithms will learn and perpetuate those biases. For example, if historical hiring data shows a tendency towards specific demographics in hiring algorithms, the algorithm may perpetuate this bias and lead to discriminatory outcomes in future hiring decisions.
James Clayton and Zoe Kleinman's paper examines how algorithms are used to make crucial decisions that influence people. The authors contend that algorithms are being employed more frequently in various fields, such as healthcare, banking, and criminal justice, and that these choices significantly impact people's lives. The use of algorithms in decision-making is discussed in the article, along with some potential advantages. Algorithms, for instance, can help increase productivity and accuracy and uncover patterns and trends that are difficult to spot with the unaided eye. However, the article also brings out some issues with the use of algorithms in decision-making. For instance, the authors contend that algorithms can be biased and can be used to target particular racial or ethnic groups for discrimination.
The article ends by urging additional studies into applying algorithms to decision-making. Before algorithms are extensively used, the authors contend that it is critical to grasp their possible advantages and risks.
As algorithms become more prevalent in decision-making, it's essential to consider the potential risks and challenges. Although algorithms can provide many advantages, we should also be mindful of the following concerns:
- When algorithms use data from the past to make decisions and predictions, they can unintentionally maintain and even worsen any biases in that data. This can result in unfair outcomes, especially in fields like hiring, lending, and law enforcement, where biased algorithms may impact certain groups more.
- In the business world, hiring processes increasingly use algorithms to improve efficiency and eliminate biases. However, it is crucial to recognise that these algorithms can inadvertently perpetuate past biases present in hiring data, resulting in discriminatory outcomes.
- Incorrect algorithms may reject highly qualified candidates or display prejudice towards specific groups, exacerbating inequalities in the job market.
Several notable articles delve into the impact of hiring algorithms on equity and bias in hiring practices. One such publication, "Help Wanted: An Examination of Hiring Algorithms, Equity, and Bias" by Aaron Rieke and Miranda Bogen, delves into predictive hiring tools' implications on fairness in hiring. The study sheds light on prevalent mechanisms used by employers and provides recommendations for comprehensive evaluations to mitigate biases.
In "All the Ways Hiring Algorithms Can Introduce Bias" by Miranda Bogen, published in the Harvard Business Review, the author explores how bias can infiltrate hiring algorithms at different process stages. The article highlights that many hiring algorithms inherently carry biases, with only a few tools showing the potential to promote equality by proactively addressing disparities.
Recent articles continue to analyse hiring algorithms' impact on hiring practices and the potential for bias. The article "Tap the Talent Your Hiring Algorithms Are Missing" from the Harvard Business Review's May-June 2022 issue addresses the challenge of employers seeking qualified candidates while overlooking potential talent. It provides recommendations for closing this gap and ensuring a fair hiring process.
Another relevant article, "AI Recruitment Algorithms and the Dehumanization Problem," published in the Ethics and Information Technology academic journal, raises concerns about the dehumanizing effects of AI recruitment algorithms in the hiring process. It emphasizes the need to be mindful of potential dehumanization while incorporating AI algorithms in recruitment.
As organizations continue to embrace algorithms to streamline operations, it is imperative to critically assess their impact and be proactive in addressing biases to ensure equitable outcomes. By fostering open discussions and staying informed about the implications of algorithm-driven decision-making, we can strive towards creating a fairer and more inclusive future.
Financial Trading
Using algorithms for trading in the financial markets has become a prevalent practice. The algorithms implemented in this system execute trades by adhering to pre-established rules and considering prevailing market conditions. While increased market efficiency is a potential benefit, it is essential to acknowledge the potential risks associated with sudden market fluctuations and flash crashes arising from trading decisions' rapid and automated nature.
Algorithmic trading, or algo-trading or automated trading, leverages advanced computer algorithms to swiftly execute numerous trades within financial markets. The algorithms employed in this system are designed to thoroughly analyse market data, effectively identify potential trading opportunities, and autonomously execute orders without human intervention. The advent of algorithmic trading has brought about substantial changes in the financial markets, offering a range of advantages and potential risks.
High-frequency trading refers to algorithmic trading in which computers execute trades in microseconds or nanoseconds. HFT companies employ sophisticated algorithms to exploit minor price differences and market inefficiencies. These algorithms can execute thousands of trades per second based on rapidly changing market conditions, like price fluctuations or imbalances in order books.
Market makers play a vital role in facilitating liquidity within financial markets. Market-making algorithms employ a continuous monitoring process to observe bid-ask spreads and make automatic adjustments to their quotes. This ensures the provision of liquidity for specific securities. These algorithms aim to generate profits by capitalising on the bid-ask spread while managing a well-balanced inventory of buy and sell orders.
Statistical arbitrage algorithms aim to detect instances of mispricing among correlated financial instruments. For example, an algorithm could analyse the price movements of two correlated stocks and execute trades when it identifies deviations from their typical relationship. The objective is to capitalise on the anticipated convergence of prices.
Momentum trading algorithms strategically capitalise on market trends and price momentum by purchasing assets exhibiting upward price movement and, conversely, selling assets that have demonstrated downward trends. These algorithms frequently utilise technical indicators and historical price patterns to identify potential trading opportunities.
Volatility trading algorithms aim to generate profits by capitalising on market fluctuations, with a specific focus on the price volatility of various assets. These algorithms adapt their trading strategies to market volatility by employing risk management techniques, including option trading strategies and dynamic hedging.
Trading algorithms that rely on news-based analysis utilise real-time news sources, social media platforms, and market sentiment to identify potential market-moving events. These algorithms can respond automatically to news releases and adjust trading positions.
Algorithmic trading allows trades to be executed at speeds and frequencies impossible for human traders. This results in improved market efficiency and liquidity. Additionally, algo-trading can efficiently conduct trades, which helps to lower transaction costs and benefits investors. Algorithms ensure that trades are made according to predefined rules, eliminating the potential for human emotional biases that may lead to irrational trading decisions.
There are various risks associated with algorithmic trading, including the possibility of malfunctioning algorithms or technical glitches that can cause sudden market disruptions or flash crashes, as was witnessed during the 2010 "Flash Crash" that temporarily wiped off trillions of dollars from global stock markets. Additionally, unscrupulous actors can exploit sophisticated algorithms to manipulate prices or create artificial market movements for their benefit. Furthermore, algorithms trained solely on historical data might not be able to anticipate unforeseen market events or sudden changes in market conditions. Moreover, regulators face challenges overseeing and monitoring algorithmic trading, ensuring fair and orderly markets, and dealing with potential market abuses.
Implementing algorithmic trading has significantly enhanced the efficiency and liquidity of financial markets. However, it is essential to note that certain risks and challenges are associated with this situation that requires careful monitoring, regulation, and management to uphold market integrity and stability.
Predictive Policing
In certain regions, law enforcement agencies utilise algorithms to forecast areas with high crime rates and distribute resources accordingly. Relying too heavily on algorithms can result in biased and discriminatory policing practices. This is because these algorithms are typically trained using historical crime data, which may already contain biases present in law enforcement.
Recently, there have been discussions on the possibility of bias in the algorithms utilised in the criminal justice system. A SpringerLink article from 2022 highlights the potential for prejudice and discrimination in using artificial intelligence by criminal courts. The article analyses how these systems contribute to societal bias and discrimination and offers potential alternative approaches to the current predictive justice mechanisms in use.
The article titled "Criminal justice algorithms: Being race-neutral doesn't mean race-blind" discusses the issue of racial bias in criminal justice algorithms. The publication date of the article is March 31, 2022.
The article explores using an algorithm known as PATTERN (Prisoner Assessment Tool Targeting Estimated Risk and Needs) to predict recidivism rates within prison populations. The algorithm mentioned is a key component of the First Step Act, passed by Congress in 2018 and received substantial bipartisan support. The First Step Act aims to reduce certain criminal sentences and enhance prison conditions. One of the changes includes a reward system for federal inmates, where they can be granted an early release if they actively participate in programmes specifically designed to minimise their chances of committing another offence.
In December 2021, the Department of Justice reviewed the PATTERN system, revealing that it tends to overpredict recidivism rates for minority inmates by 2% to 8% compared to white inmates. Critics are concerned that PATTERN perpetuates racial biases that have historically plagued the U.S. prison system. The article's authors argue that to ensure equal accuracy for all inmates, the algorithm used in PATTERN may need to consider the inmates' race. In essence, it may be necessary to emphasise race more than less to attain equitable outcomes for different racial groups. This apparent paradox is often observed in discussions surrounding fairness and racial justice.
In a 2020 New York Times article, predictive algorithms in the criminal justice system are explored, focusing on the potential for these algorithms to be biased. The article explains that these algorithms use historical data to predict future crimes, but if this data is biased, the predictions may be inaccurate and unfair. For example, suppose the data used to train the algorithm is disproportionately represented by certain groups, such as people of colour or low-income individuals. In that case, the algorithm may exhibit bias against these groups.
In order to ensure that the criminal justice system is fair and just, it is imperative to thoroughly design and rigorously test all algorithms utilised within it. It is essential to acknowledge the potential sources of bias and discrimination within these algorithms and take proactive measures to mitigate them. By doing so, it is possible to create algorithms that benefit all individuals, regardless of their background or circumstances. These articles underscore the critical importance of this process and highlight the need for continued efforts to increase transparency and accountability within the criminal justice system.
Autonomous Vehicles
Self-driving and autonomous vehicles depend on advanced algorithms to effectively navigate and make instantaneous decisions while operating on the road. Although technology can potentially enhance road safety, excessive dependence on algorithms can result in accidents or unanticipated behaviours in intricate and unpredictable driving scenarios.
Recently, there has been an investigation regarding Tesla and the National Highway Traffic Safety Administration (NHTSA). This is due to a fatal crash in California in 2018. It is believed that Tesla's self-driving technology may have played a role, and as a result, the NHTSA has been investigating over 20 fatalities since 2016. Specifically, the incident involving a Tesla Model 3 is currently being scrutinised. Reuters said the driver may have used one of Tesla's advanced driver assistance systems during the accident. The NHTSA is not the only organisation investigating Tesla's self-driving technology. The Department of Justice and the California DMV also conduct their inquiries. The investigation by NHTSA is still ongoing, so it's uncertain what the final results will be. Nonetheless, the analysis suggests that NHTSA is concerned about the safety of Tesla's Autopilot and FSD features.
The agency has initiated several investigations concerning Tesla vehicles, and it is apparent that the NHTSA has concerns about potential risks associated with the technology. It is a timely reminder that autonomous driving technology is still in development. As such, it is vital to approach its research and development with caution and responsibility. This investigation highlights the importance of regulating autonomous driving technology. There is a gap in safety oversight due to the need for federal regulations governing autonomous vehicles. Therefore, as this technology evolves, we must establish robust regulations to ensure public safety.
Health Diagnosis and Treatment
Although algorithms have demonstrated potential in healthcare, it is essential to acknowledge the risks associated with their utilisation in the context of diagnosis and treatment decisions. Misdiagnosis or inappropriate treatment recommendations may occur if a medical algorithm is trained using biased or limited data. In healthcare settings, it is crucial to prioritise patient data privacy and security when utilising algorithms.
Research has shown that there is evidence of racial bias present in healthcare algorithms. One particular study published in Science in 2019 brought to light significant racial discrimination in a commonly used healthcare algorithm. This algorithm relied on historical healthcare spending as a measure of health needs. However, it failed to take into account that black patients often receive less healthcare spending compared to white patients with comparable health needs. As a result, this algorithm underestimated the health needs of black patients, which could lead to incorrect predictions and treatment recommendations.
Numerous peer-reviewed studies have examined the potential biases of algorithms in healthcare. In a review published in PLOS Digital Health in 2023, the authors discuss the various sources of preference that can influence the development of AI algorithms in healthcare. They examine each process step, including problem framing, data collection, preprocessing, development, validation, and implementation. A recent study published in PLOS Digital Health in 2022 examines the use of AI in clinical medicine and aims to identify any disparities related to population and data sources.
In a widely recognised case, an algorithm employed in a healthcare system to propose treatment plans for cancer patients resulted in inappropriate recommendations for specific individuals. The algorithm did not consider crucial factors, such as patient preferences, medical history, and particular characteristics of the cancer. Consequently, certain patients were provided with treatment plans that may have needed to be more suitable for their circumstances, which could have resulted in adverse effects and less-than-optimal outcomes. This highlights the importance of thoroughly validating and consistently updating medical algorithms to ensure that they offer suitable and personalised treatment recommendations.
Here are some crucial points about bias from an article titled "Addressing Bias: Artificial Intelligence in Cardiovascular Medicine," published in The Lancet Digital Health.
Algorithmic bias can have a significant impact on cardiovascular medicine. Biased data used to train algorithms could be a contributing factor, as it may not contain an equitable representation of patients from all racial and ethnic backgrounds. This could lead to less accurate algorithms for underrepresented groups.
Algorithmic bias can also arise when inappropriate or irrelevant features are utilised in the algorithm. An algorithm used to predict the risk of a heart attack may include additional factors not typically associated with the risk of a heart attack, such as the patient's zip code. This can result in algorithms that are less accurate and potentially harmful.
Algorithms have the potential to develop bias depending on their usage. For example, an algorithm that is utilised to determine patient care can be applied in a manner that is unjust towards patients who belong to marginalised groups.
Addressing algorithmic bias in cardiovascular medicine poses several challenges. One of the main obstacles is the need for more available data on patients from underrepresented groups. Creating algorithms that cater accurately to all patients can also be tricky, especially when identifying and eliminating irrelevant features. This is because the features associated with a disease's risk may not be noticeable. Additionally, ensuring that algorithms are used fairly and equitably can be challenging since they can be applied in different ways, and it can sometimes be confusing how to use them reasonably for all patients.
A primary healthcare institution recently experienced a data breach that compromised the personal health information of thousands of patients. The breach involved data utilised for training and updating medical algorithms within the institution. As a result, the sensitive medical records of patients were compromised, leading to significant concerns regarding data privacy and security in healthcare settings. This incident highlights the crucial significance of protecting patient data and implementing strong security measures when using algorithms in healthcare. These measures are essential to prevent possible breaches and safeguard patient privacy.
In conclusion, although algorithms show potential in healthcare for diagnosis and treatment decisions, it is crucial to address the associated risks. Instances of bias, inappropriate treatment recommendations, and data privacy breaches highlight the necessity of thorough data validation, continuous algorithm evaluation, and robust security protocols. These measures ensure patient information's reliability, accuracy, and ethical handling in medical algorithms.
Credit Scoring and Loan Approval
A lot of financial institutions utilise algorithms as a means to assess a person’s creditworthiness and grant loans. While this may seem efficient, over-reliance on these algorithms can lead to unjust denial of credit or loans for specific individuals, particularly those with a lengthy credit history.
Financial institutions rely on credit scoring and loan approval algorithms as vital tools to evaluate the creditworthiness of individuals and make informed decisions regarding loan applications. These algorithms utilise a range of data points and statistical models to assess an individual's credit history, income, debt-to-income ratio, and other pertinent factors to forecast their ability to repay a loan. Here is a comprehensive overview of credit scoring and loan approval algorithms, highlighting their advantages and potential drawbacks. Additionally, we will discuss specific incidents that have raised concerns in this field.
With the help of algorithms, data analysis can be done swiftly, resulting in quicker loan processing and more efficient decision-making than manual evaluations. This leads to objective and efficient decision-making. Using algorithms to evaluate loan applications ensures that objective criteria are applied consistently, reducing the risk of biased or discriminatory decisions based on subjective factors. By utilising past data and predictive modelling, algorithms can more effectively evaluate credit risk, resulting in more precise decisions on loan approvals. Using credit scoring algorithms gives people with limited credit histories more opportunities to establish credit and access financial services. This can significantly benefit their financial standing. Algorithms minimise human errors and subjective judgement that occur during manual evaluations.
There are potential risks associated with credit scoring and loan approval algorithms. If the historical data used to train these algorithms is based on discriminatory lending practices, it can unintentionally perpetuate bias. This can result in certain demographic groups having unequal access to credit.
Understanding how specific algorithms assess creditworthiness can be difficult for consumers, especially when they are highly complex and need more transparency. When personal and sensitive data is used in algorithms, privacy concerns arise if proper protection measures are not in place. During economic downturns, algorithms may make inaccurate predictions as they may not account for current financial or situational changes.
If fraudsters or malicious actors take advantage of weaknesses in the algorithm or input inaccurate data, it could result in unjustified loan approvals or rejections. In 2016, Wells Fargo committed an unethical act that rocked the banking industry. The Wells Fargo account fraud scandal involved employees opening accounts and credit cards in customers' names without their consent to meet sales targets. As a result of this scandal, the bank was fined $185 million, and the CEO, John Stumpf, resigned. This scandal also raised concerns about the bank's ability to evaluate the creditworthiness of its customers. The bank's failure to properly assess their customers' creditworthiness was evident in their opening of accounts without their consent and subsequent fines.
Studies have revealed that algorithms banks and other financial institutions use may be biased. In 2018, the National Bureau of Economic Research conducted a survey that indicated that mortgage lenders' algorithms were racially discriminatory. This resulted in African American and Hispanic borrowers being charged higher interest rates than white borrowers with similar credit scores.
In 2019, the National Bureau of Economic Research published a working paper titled "Consumer-Lending Discrimination in the FinTech Era." The study analysed the possibility of bias in mortgage lending by FinTech lenders, who use algorithms to make lending decisions. The results show that although FinTech lenders do not discriminate based on race or ethnicity when approving loans, they charge higher interest rates to African American and Hispanic borrowers than to white borrowers with similar credit profiles. These findings suggest that algorithms may display bias, emphasising the importance of designing and testing algorithms carefully to ensure they are fair and unbiased.
Tax Administration
Tax administrations worldwide have been actively investigating the potential of Artificial Intelligence (AI) and machine learning (ML) technologies to enhance their capabilities in preventing and detecting instances of tax evasion and tax fraud. Implementing these technologies can augment the risk-management and business rule-matching methodologies employed by tax agencies for conducting background checks, assessing eligibility, and identifying fraudulent activity. Artificial intelligence and machine learning technologies can promptly and proactively address identified events by leveraging near-real-time data. These technologies can effectively integrate multiple data sources and facilitate sharing within the tax ecosystem. Furthermore, they can enhance our understanding of taxpayer reporting and compliance behaviour, improve data quality, and enable more proactive digital audits. The Australian Taxation Office (ATO) has developed ANGIE (Automated Network & Grouping Identification Engine), an artificial intelligence system designed to identify patterns of interest within intricate corporate structures and high-wealth individuals aiming to evade tax obligations.
ANGIE – the automated network and grouping identification engine – will allow the task force to visualise and analyse "large and complex networks of relationships."
The Australian Taxation Office (ATO) has worked with various third-party organisations to create ANGIE (Automated Network & Grouping Identification Engine). In 2019, McKinsey, a strategy consulting firm, conducted a high-level design of ANGIE. The ATO has also incorporated a graph database from TigerGraph, a US-based startup, into its newly developed big data platform. The solution is supported by utilising a graph database, which represents intricate networks by storing data about entities (nodes) and their interconnections (edges).
TigerGraph is a company that offers graph database technology to different organisations, such as the Australian Taxation Office (ATO). ATO uses it for their ANGIE (Automated Network & Grouping Identification Engine) solution. TigerGraph is utilised by various organisations, including China Mobile, the biggest mobile service provider globally. They use TigerGraph to detect phone fraud in real-time by analysing the calling patterns of their pre-paid subscribers. China Mobile is among the numerous organisations taking advantage of TigerGraph to enhance their operations. TigerGraph has garnered favourable feedback from users in the Cloud Database Management Systems industry, achieving a rating of 4.6 stars on Gartner Peer Insights based on 48 reviews.
Does the above information clarify the functioning of ANGIE (Automated Network & Grouping Identification Engine)?
The transparency level may vary based on one's viewpoint. While the ATO has provided some information on ANGIE (Automated Network & Grouping Identification Engine), it is ultimately up to the user to determine if the disclosed details are sufficient for their needs.
Tax departments employ a range of algorithms to enhance their operational efficiency and overall effectiveness. EY has been at the forefront of developing explainable AI. This technology incorporates analytics to provide insights into the decision-making process of algorithms, thereby offering transparency in determining outcomes. The AI system analyses the transaction description and vendor information when categorising transactions and allocating them to tax categories. Artificial intelligence (AI) systems can enhance the accuracy of tax forecasting through the utilisation of predictive analysis. Algorithms can identify sales trends annually, monthly, or even more frequently. Artificial intelligence algorithms can leverage weather patterns to analyse the potential impact of climate change on sales within specific regions.
The Australian Taxation Office (ATO) leverages data and analytics to get deeper insights and provide the Australian population with high-quality services. They create better, quicker, smarter decisions, give the results agility, advise the government, and influence what they do for the community by using data and analytics to understand their clients better and improve their relationships. For instance, information submitted to the ATO by third parties like banks, health funds, and governmental organisations is utilised to pre-fill an individual's annual tax return. The ATO pre-filled about 85 million data in 2020 using data and analytics technology.
Using data analytics for tax purposes poses potential risks and issues that companies should be aware of. Tax authorities increasingly rely on data to make compliance and audit determinations, which they share with other jurisdictions. They may face risks if a company's people, processes, and systems need to be updated or in line with government requirements. However, tax authorities should address algorithmic bias, unintended consequences, lack of transparency, and overreliance on algorithms. Tax authorities must use data and insights to embed ethical practices in their corporate culture and values.
It is essential to ensure transparency in AI engines like ANGIE for detecting tax fraud. Failure to do so could lead to various consequences, including:
AI engines must offer transparency to tax authorities and investigators to guarantee their conclusions are easily understandable. With this transparency, decisions made by AI may be perceived as manageable. There is no accountability for the algorithm's findings; defending or challenging them can be difficult. This can lead to legal disputes and erode public trust.
It is crucial to prioritise establishing transparency in AI engines and their algorithms to identify and rectify any potential biases effectively. Failure to address this issue may inadvertently perpetuate biases in detecting tax fraud, unjustly targeting specific individuals or groups.
Insufficient transparency can challenge tax authorities to verify the accuracy and dependability of AI-generated outcomes. This scenario may lead to instances where tax fraud cases are overlooked, or false positives are generated, resulting in the inefficient allocation of resources and impeding the effectiveness of tax enforcement efforts.
If artificial intelligence functions in an opaque manner, it may result in individuals needing more awareness regarding the utilisation and analysis of their data. The absence of transparency in this matter may give rise to privacy concerns, mainly if sensitive personal information is being processed without explicit consent or comprehension.
Transparency is essential for sustaining public trust in tax administration operations. If artificial intelligence operates as a black box, public confidence in the tax system may erode, leading to cynicism and resistance to tax compliance.
In areas with strict rules surrounding algorithmic decision-making, utilising AI decisions without transparency may result in legal disputes and failure to comply with data protection regulations.
Transparency in AI algorithms is of utmost importance to effectively identify and address weaknesses or flaws. Without understanding how the system works, improving and optimising fraud detection accuracy can be daunting.
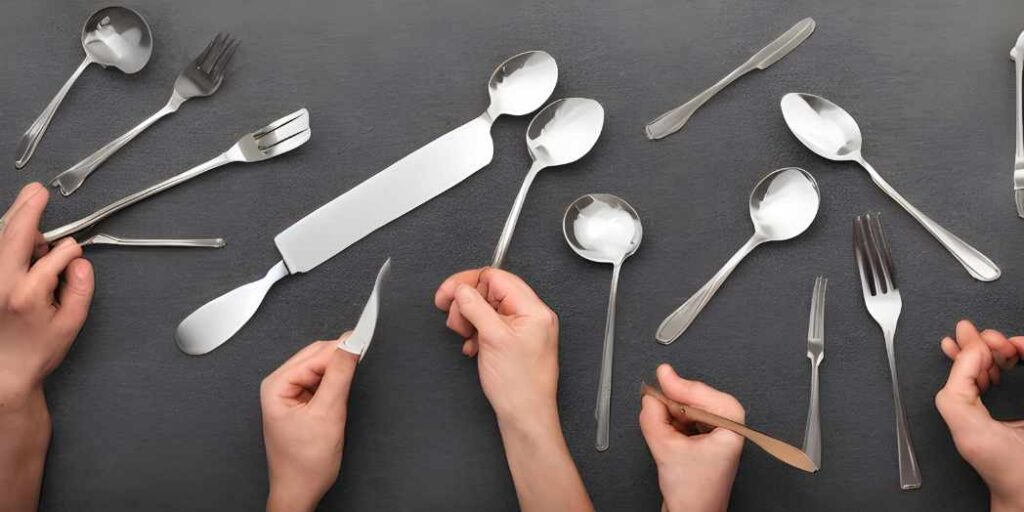
Image designed by Deepai
Is the Genie or Jinni out of the bottle, or are they still contained? Legend has it that Jinni have the ability to see four seconds into the future, giving them an edge over humans. However, even with modern technology, AI must improve accuracy to predict which piece of cutlery a person will reach for first.
Transparency needs to be a core principle in AI design to prevent these unintended outcomes. The algorithm's logic, data sources, and potential biases should all be transparent to the tax authorities. AI's efficacy and fairness in detecting tax fraud while adhering to ethical and legal norms can be ensured by regular audits and independent assessments of its performance. Further, the public's trust and confidence in AI's involvement in tax enforcement can be bolstered by open communication and education regarding the technology's application.
What's Next
In today's world, algorithms are ubiquitous, leading some to question the authenticity of our reality. While we cannot definitively answer this question, algorithms are used to manipulate and regulate various aspects of our lives. From traffic lights to targeted advertisements and news selection, algorithms control our environment.
Algorithms are taking over
Do you feel like algorithms are taking over and controlling our every move? We seem surrounded by these complex codes, always being watched and manipulated without realising it.
On the other hand, there is no evidence suggesting that we live in a simulated reality. The algorithms we use are based on real-world data, and they cannot create a perfect simulation. Additionally, algorithms, such as the experience of consciousness, cannot explain many aspects of our reality.
Ultimately, whether we live in a simulated reality is a philosophical debate. There is no scientific evidence to prove or disprove the existence of simulated realities. However, the prevalence of algorithms in our surroundings does raise some interesting questions about the nature of reality.
"The only way to escape algorithms' influence is to disconnect the power source or remove the batteries"
In conclusion, it is crucial that we thoroughly examine and discuss the use of algorithms in decision-making processes that have a significant impact on people's lives. This issue is multifaceted and requires careful consideration and critical thinking as we navigate the rapidly evolving technological landscape. Our primary objective should be maximising algorithms' benefits while minimising any potential harm they may cause.
We are preparing an upcoming article that delves into the intricate topic of algorithms and their involvement in determining the appropriate time to end human life. Our article will examine the ethical implications and technological advancements that impact this complex issue. We hope you stay tuned for this insightful conversation.
Some of the information in this article might be linked to archived articles and could use an update. To ensure complete accuracy, please verify the time stamp on the linked pages to validate the most recent update.